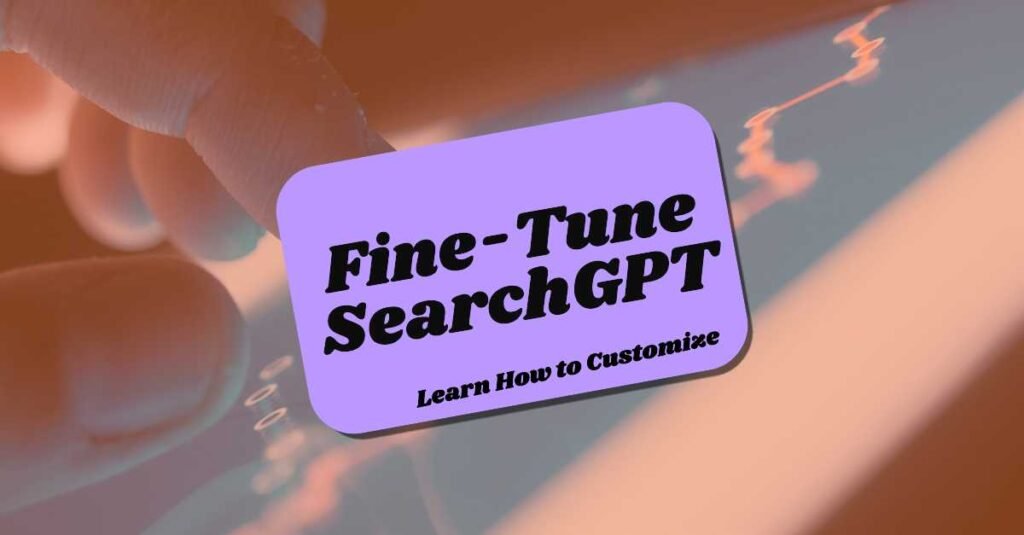
Introduction
Fine-tuning SearchGPT means adjusting its capabilities to match your specific goals, whether for a business or personal use. This involves training the model on specific datasets relevant to your field and tweaking its settings to improve how it responds. This way, you can get more relevant and accurate results that make your interactions better.
SearchGPT is a powerful tool that can generate answers using a large amount of data and perform accurate search tasks. While it works well from the start, users can make it even better by customizing it to fit specific industries, topics, or personal needs. Fine-tuning SearchGPT helps unlock its full potential, providing more focused responses based on expert knowledge.
Customizing SearchGPT means changing how it behaves by adjusting its settings or training it with specific information. This improves how well it performs and makes the tool interact with users in a more personalized way.
In this guide, we’ll take a closer look at the fine-tuning process. We’ll walk you through each step, from selecting the right dataset to adjusting important settings and fixing common problems. We aim to help you make SearchGPT work best for you. Let’s begin this journey to customize SearchGPT for your needs.
Table of Contents
What is Fine Tuning?
Fine-tuning is the process of refining an AI model to make it more accurate for specific tasks. It involves adjusting the model using additional, focused data to improve its performance in particular areas. By training the model with new, relevant information, fine-tuning allows it to deliver better, more precise responses that match the needs of certain industries or users. This process enhances the overall efficiency of the AI, ensuring it provides tailored results for specific tasks or fields.
Key Steps:
- Data Collection: Gather specific, relevant data for training.
- Model Adjustment: Modify the model’s parameters for the task.
- Training: Use the data to teach the model new skills.
- Evaluation: Test and measure the model’s performance after training.
Why Fine-Tune SearchGPT?
Fine-tuning SearchGPT allows users to improve the model’s performance for specific needs, making it more accurate and relevant to particular industries or tasks. It helps deliver customized responses, ensuring the AI aligns with targeted expertise and provides better results.
There are several benefits to fine-tuning SearchGPT, particularly for businesses and specialized applications:
- Improved Relevance: By customizing the dataset, you ensure that SearchGPT generates results aligned with specific industries, such as healthcare, finance, or legal.
- Personalized User Experience: Fine-tuning enables the model to respond in a personalized tone that resonates with your brand or audience.
- Enhanced Performance for Niche Queries: General models may not adequately handle niche or specialized queries. Fine-tuning ensures precise results in specialized fields like scientific research or legal documentation.
- Optimized Efficiency: Fine-tuning improves both the response speed and accuracy of SearchGPT, resulting in higher engagement.
- Reduced Noise: A standard SearchGPT may return a wide range of results. Fine-tuning narrows down the scope, delivering precise answers and minimizing irrelevant outputs.
Can Fine-Tuning Be Applied to SearchGPT?
Yes, you can fine-tune SearchGPT. Fine-tuning involves training the model on specific datasets to improve its performance for particular tasks or topics. This process allows the model to generate more accurate and relevant responses tailored to your needs.
Steps for Fine-Tuning SearchGPT
Fine-tuning SearchGPT involves a series of steps to enhance its performance for specific tasks. First, gather relevant data that aligns with the desired application. Next, adjust the model’s parameters to suit the task’s requirements. Then, train the model using the collected data, allowing it to learn patterns and improve accuracy.
Finally, evaluate the model’s performance through testing to ensure it meets expectations. This process helps create a more tailored and effective version of SearchGPT for particular use cases.
Fine-tuning SearchGPT requires a clear understanding of your goals and systematic steps to ensure effectiveness.
Step 1: Define Your Objectives
Understand what you want from the model: is it to generate more accurate responses, or do you need specific tones or styles? Setting clear objectives will guide the rest of the fine-tuning process.
Step 2: Choose or Prepare the Right Dataset
Choose a relevant dataset that aligns with your objectives. If your aim is to fine-tune SearchGPT for financial analysis, include market reports and historical financial data in your dataset.
Step 3: Train the Model
Use the prepared dataset to train SearchGPT. Run training cycles, which will allow the model to integrate the nuances and intricacies of your data.
Step 4: Test and Validate
Once training is complete, conduct rigorous testing to ensure the model’s output meets expectations. If results are unsatisfactory, adjust the dataset or retrain the model.
Step 5: Deploy the Fine-Tuned Model
After validating the model, deploy it through API integration or any desired platform. Ensure that the model is ready to serve end-users effectively.
How to Customize SearchGPT
Choosing the Right Dataset
The quality and relevance of the dataset play a key role in the success of the fine-tuning process. Here are tips for selecting a dataset:
- Relevance: Ensure the dataset reflects the specific field you want to focus on. For instance, a medical application should incorporate medical journals, clinical studies, and diagnostic guidelines.
- Diversity: A diverse dataset allows the model to handle a wide range of queries and offers more generalization for different contexts.
- High-Quality Data: Clean, error-free data is essential for accurate training. Avoid datasets with missing or inconsistent information.
- Ethical Sourcing: Use ethically sourced data to avoid biases and ensure the AI produces balanced, fair outputs.
Training SearchGPT with Specific Objectives
Training SearchGPT with specific goals helps improve its performance for certain tasks. By choosing the right data and adjusting its settings, the model can produce more accurate answers. Focusing on key objectives ensures the AI meets specific needs and provides better, more relevant responses. This also helps make the content more visible on search engines, increasing the chances of reaching the right audience effectively.
Training SearchGPT can be done in two ways: data training and parameter adjustment.
- Data Training: Feed specific datasets into the model to train it for generating accurate responses. For example, a law firm can train SearchGPT using legal documents, court rulings, and case studies.
- Parameter Adjustment: Fine-tune the internal settings of SearchGPT to manage aspects like creativity, specificity, and response length. Adjusting parameters like temperature can modify the variability of the generated output.
Important considerations for training:
- Training Time: Depending on the dataset size and complexity, training times will vary.
- Continuous Updates: Fine-tuning should be a regular process, with periodic retraining based on new data.
- Computational Resources: Ensure access to sufficient GPU or cloud resources for training.
Adjusting Search Parameters
Another method to customize SearchGPT is by adjusting its search parameters. These can be modified without full model retraining:
- Temperature: Lower temperature means more focused and deterministic outputs, while higher temperature yields creative and varied responses.
- Max Tokens: This controls the length of the response. Limiting token count can generate concise, direct answers.
- Top-P Sampling: Helps control the diversity of word choices. A lower top-p leads to more conservative responses, while a higher value allows for a wider variety of outputs.
Testing and Validating Customization
Testing and validating the customization of SearchGPT is crucial to ensure its effectiveness. After fine-tuning, implementing specific practices will help assess performance and identify areas for improvement. Here are three key practices to follow:
- Diverse Queries: Test the model with various types of questions to ensure it performs consistently across different scenarios and topics.
- Real-World Simulations: Use practical examples that users might encounter to evaluate how well the model functions in real-life situations.
- User Feedback: Collect insights from end-users and stakeholders to identify strengths and weaknesses, guiding future enhancements for better performance.
Use Cases for Customizing SearchGPT
Customizing SearchGPT has many uses in different fields. In customer support, it helps answer common questions quickly, making customers happier. In healthcare, it provides reliable information about symptoms and treatments for patients. For education, it gives personalized help to students by answering their questions. In e-commerce, it helps customers find products and make better choices, improving their shopping experience. These examples show how useful and flexible the model can be.
Customizing SearchGPT through fine-tuning has numerous use cases across various industries:
- Customer Support: A company can fine-tune SearchGPT to answer frequently asked questions in a personalized tone, improving response times and customer satisfaction.
- Healthcare: Doctors and medical professionals can use fine-tuned SearchGPT to answer complex medical queries based on diagnostic data and medical reports.
- Legal Sector: Law firms can fine-tune SearchGPT to provide accurate legal advice based on court precedents, case law, and statutes.
- Content Creation: Marketers and content creators can fine-tune the model to generate blog posts, social media content, or reports tailored to specific niches.
Best Practices for Fine-Tuning
Practicing best practices for fine-tuning is crucial to maximize the effectiveness of the model. It leads to improved accuracy and better alignment with user needs. Following systematic approaches allows for enhanced performance and reduced errors, ensuring the AI can handle specific tasks efficiently and effectively, ultimately providing more valuable insights and responses.
Best practices for fine-tuning are essential because they ensure that the model performs optimally for specific tasks. By following these practices, users can:
- Improve Accuracy: Proper techniques help the model generate more precise and relevant responses.
- Enhance Efficiency: Streamlined processes save time and resources during training and implementation.
- Meet User Needs: Tailored adjustments ensure that the model aligns with user expectations and industry requirements.
- Reduce Errors: Systematic testing and validation minimize the risk of inaccuracies, leading to better overall performance.
Practicing these methods ultimately leads to a more effective and reliable AI model.
To achieve optimal results, follow these best practices during fine-tuning:
- Clarify the Goal: Define your fine-tuning objective before starting the process.
- High-Quality Data: Ensure the dataset is free from errors and inconsistencies.
- Avoid Overfitting: Do not overtrain the model on a limited dataset to avoid poor generalization.
- Monitor Results: Continuously monitor performance after deployment to ensure the fine-tuned model delivers consistent results.
Token Limits and Cost Estimation
Token limits vary based on the AI model chosen, affecting both inference context length and training examples. For instance, models like GPT-3 have a maximum context length of 4,096 tokens, meaning they can process up to that number of tokens in a single input. This includes both the prompt and the response.
In contrast, more advanced models may offer extended limits, accommodating longer interactions. When estimating costs, consider that pricing often depends on the number of tokens processed. Higher token usage in training or inference can lead to increased costs, so it’s important to balance the complexity of inputs with budget constraints. Understanding these limits helps optimize both performance and expenses in AI applications.
Conclusion
Fine-tuning SearchGPT offers businesses and individuals the ability to customize AI to their exact needs, making it an invaluable tool for specialized applications. Whether for customer support, financial analysis, legal research, or content generation, fine-tuning enhances SearchGPT’s ability to deliver relevant, personalized results, ensuring better user engagement and efficiency.
By following the steps and best practices outlined in this guide, you can effectively fine-tune SearchGPT to maximize its performance and ensure it aligns perfectly with your objectives.
Also Read: